AI in Industry 5.0: Unlocking Manufacturing’s Full Potential
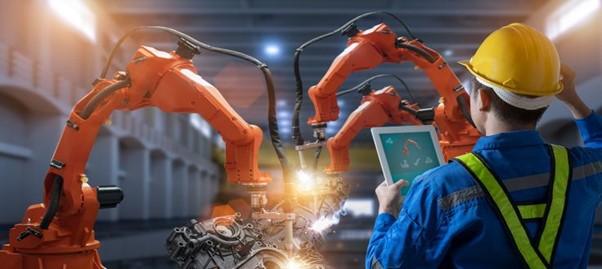
“MarketWatch predicts a 50%+ surge in AI adoption across manufacturing by 2027.”
“We’re living in an age of rapid technological change—the dawn of Industry 5.0!—where AI isn’t just about automation but about creating a smarter, more sustainable future.
AI is revolutionizing manufacturing and many other areas of our lives! In the manufacturing sector, AI is driving improvements in efficiency, quality, and safety. Here’s how:
INDUSTRY 5.0: The Future of Manufacturing
Welcome to Industry 5.0—the next industrial revolution! This isn’t just about more automation; it’s about putting humans at the heart of manufacturing. Imagine a future where factories are not just efficient but also sustainable and resilient, prioritizing human well-being and societal goals.
Key Characteristics of Industry 5.0
- Human-Centricity: Emphasizing collaboration between humans and intelligent machines. Workers are not replaced but augmented by advanced technology, enabling them to focus on more creative and strategic tasks.
- Sustainability: ensuring manufacturing processes and products are environmentally friendly. This includes reducing waste, conserving energy, and using sustainable materials to create a greener industry.
- Resilience: building systems that can withstand disruptions. This involves adopting flexible production methods and incorporating redundancy to maintain operations during unforeseen events.
The Basic Understanding
Industry 5.0 goes beyond traditional industrial goals of efficiency and profitability. It seeks to align manufacturing with broader societal values, creating workplaces that are not only productive but also ethical and inclusive. This new era promises a brighter future for all, but we’re still in the early stages of understanding its full potential.
As we explore and embrace Industry 5.0, it’s an exciting journey towards a future where technology and humanity harmoniously coexist to create a better world.
Why industry 5.0? so here is the one for your question
Traditional manufacturing is hitting a wall. High-volume production struggles with customization and innovation. Long lead times and hefty setup costs hinder flexibility. Plus, the environmental impact is a growing concern. Industry 4.0 brought automation, but what if there was a smarter way? Enter AI-powered manufacturing…

AI in Manufacturing: From Concept to Reality
Forget endless design tweaks and time-consuming simulations! AI is revolutionizing manufacturing. Generative AI tools are churning out countless design options and running simulations in a flash, leading to faster product development, increased experimentation, and the ability to create truly personalized products. Imagine a factory floor where humans and robots collaborate seamlessly, boosting efficiency, predicting equipment failures, and minimizing downtime. AI also optimizes supply chains by forecasting demand and streamlining logistics. While challenges like data security exist, the potential for increased productivity and innovation is undeniable. Manufacturers embracing AI are poised to lead the way in the exciting new era of Industry 4.0.
Benefits of Using AI in Manufacturing
Increased Efficiency
- Accelerated production
- Reduced human error
- Optimized workflows
- Streamlined processes
- Minimized manual intervention
Cost Reduction
- Reduced labor and maintenance expenses
- Lowered waste and optimized energy consumption
- Leaner and more cost-effective production
Improved Decision-Making
- Real-time data processing
- Informed and data-driven decisions
- Risk minimization through simulations
Increased Safety
- Cobot collaboration for hazardous tasks
- Smart systems and AR-guided workflows
- Minimized risks to human employees
Sustainability
- Optimized resource allocation
- Reduced energy use and waste
- Minimized maintenance needs
Innovation and Competitive Advantage
- Faster prototyping and generative design
- Digital twin simulations
- Reduced time-to-market
- More advanced product designs
- Enhanced competitiveness
AI is truly shaping the future of manufacturing, bringing a host of benefits that are transforming how we produce goods and innovate.
INTRODUCTION TO INDUSTRY 5.0
Industry 5.0 signals a new wave of global industrial transformation. Unlike its predecessors, this era places human well-being at the core of manufacturing systems, aiming to achieve social goals beyond just employment and growth, ensuring sustainable development for all humanity. Currently, Industry 5.0 is still in its infancy, with limited and unsystematic research findings. This article reviews the evolution of Industry 5.0 and its three primary characteristics: human-centricity, sustainability, and resilience, while also discussing its underlying principles.
Challenges in Traditional Manufacturing and Industry 4.0
Manufacturing industries and investors consistently seek to improve techniques to reduce costs, conserve energy, and expand capabilities. However, traditional manufacturing faces numerous challenges:
- High-Volume Production: Struggles to produce smaller batches or highly customized items.
- Design Limitations: Limited in creating complex shapes, hindering innovative designs.
- Long Lead Times: Tooling and setup slow down production, making it hard to adapt to market changes.
- High Costs: Setup and maintenance costs can be prohibitive for smaller businesses.
- Environmental Impact: High energy consumption and waste generation contribute to environmental concerns.
How AI Can Address Challenges and Drive Industry 5.0
Technological growth is reshaping our lives, making them smarter and more convenient. Concepts like Smart Society 5.0, Healthcare 5.0, and Agriculture 5.0 exemplify our rapidly evolving lifestyle. The Industrial Revolution 5.0 (IR 5.0) envisions future industry development trends, focusing on prosperity beyond jobs by integrating more intelligence into daily living through cutting-edge technologies like Explainable Artificial Intelligence (XAI).
AI is transforming the manufacturing industry by:
- Optimizing Processes: From predictive maintenance for critical equipment to sophisticated algorithms for supply chain management.
- Enhancing Operational Efficiency: Real-world implementations of AI illustrate how these innovations are driving cost reductions and facilitating global expansion.
Manufacturing Transformed: How AI is Driving Innovation and Efficiency
AI is revolutionizing manufacturing by:
- Faster Product Development: Generative AI tools produce countless design options and run simulations quickly, allowing for more experimentation and customized products.
- Collaborative Work: Humans and robots working together seamlessly boosts efficiency and predicts equipment failures, minimizing downtime.
- Optimized Supply Chains: AI forecasts demand and streamlines logistics, enhancing overall efficiency.
- Addressing Challenges: Despite concerns like data security and integration, the potential for increased productivity and innovation is undeniable.
Embracing AI means fewer human resources are needed for dangerous and laborious tasks, reducing workplace accidents. Although the initial capital investment in AI for manufacturing is significant, the ROI is high, with businesses enjoying considerably lower operating costs.
Industry 5.0 is ushering in a new era of manufacturing where human-centricity, sustainability, and resilience are paramount. The integration of AI into manufacturing processes not only addresses the limitations of traditional methods but also paves the way for a more innovative and efficient future. As businesses embrace AI, they are poised to lead in this exciting new industrial revolution.
USE CASES IN MANUFACTURING
Let’s deep dive into the applications of AI in manufacturing industries.
ROBOTICS AND AUTOMATION
Robots and automation are often used interchangeably, but they have distinct meanings. Automation broadly refers to using technology to perform tasks without human intervention, encompassing everything from simple timers to complex software systems. Robotics, on the other hand, specifically focuses on the design and engineering of robots – programmable machines capable of carrying out tasks autonomously or semi-autonomously. Essentially, robotics is a subset of automation, as robots are tools used to achieve automation. While all robots automate tasks, not all automation involves robots.
ROBOTS
Robotics in manufacturing represents a transformative force in the field of engineering, revolutionizing how products are designed, produced, and delivered. The integration of robotics into manufacturing processes has led to significant improvements in efficiency, precision, and safety.
Basic Principles and Concepts
Robotics in manufacturing involves the use of automated machines, known as robots, to perform tasks traditionally carried out by human workers. These tasks can range from simple repetitive actions to complex operations requiring high precision. The key components of a robotic system include:
- Actuators: Devices that convert energy into motion, enabling the robot to move and interact with its environment.
- Sensors: Instruments that gather data about the robot’s surroundings, allowing it to make informed decisions.
- Controllers: Systems that process sensor data and send commands to the actuators, coordinating the robot’s actions.
- End Effectors: Tools or devices attached to the robot’s arm, designed to interact with objects (e.g., grippers, welding torches).
Classification of Robots Based on Application
- Industrial Robots: Used in various manufacturing processes such as welding, painting, assembly, pick and place operations, packaging, labeling, palletizing, product inspection, and testing.
- Non-Industrial Robots: Used in other sectors like healthcare, logistics, and domestic applications.
As we delve deeper into these applications, it’s clear that AI-driven robotics and automation are key drivers in the evolution of modern manufacturing.
Read- Agricultural robots for field operations: Concepts and components – ScienceDirect
Automation in Manufacturing
Automation is poised to elevate the manufacturing industry to unprecedented levels of accuracy and productivity, surpassing human capabilities (Haskovic et al., 2018). It can operate in environments that are hazardous, monotonous, or complex for humans. Future robotics are expected to have advanced capabilities like voice and image recognition to replicate intricate human tasks.
Examples of Automation and AI Integration
- Internet of Things (IoT): Enables the automation of physical devices.
- Artificial Intelligence (AI): Enhances automation by making intelligent decisions. For instance, a smart thermostat (an IoT device) uses AI to learn user preferences and adjust the temperature accordingly.
Case Study: Schneider Electric Schneider Electric employs a variety of robots to enhance their manufacturing processes. Here are some examples:
- Lexium SCARA Robots: These Selective Compliance Articulated Robot Arms (SCARA) are designed for high-speed, precision tasks. They are used in electronics assembly, battery manufacturing, logistics, food and beverage, and life sciences for applications such as assembly, pick-and-place, inspection, dispensing, coating, and welding.
- Lexium MAX: High-performance industrial robots used for heavy-duty tasks in various industries.
- Lexium TAS, PAS, PAD, CAS, CAR: These robots are tailored for specific applications like transport, assembly, packaging, and automation control.
- Lexium MC12 Multi-Carrier Transport System: This system is utilized for transporting materials within manufacturing facilities, enhancing productivity and flexibility.
These robots help Schneider Electric optimize production lines, improve efficiency, and ensure high-quality output. Automation and AI integration are crucial for driving innovation and efficiency in manufacturing, enabling companies to stay competitive in an ever-evolving market.
Read: Integrated SCARA Robots Redefine Precision | Schneider Electric
SUPPLY CHAIN MANAGEMENT
Supply chain management plays a crucial role in the manufacturing industry, and artificial intelligence has emerged as a game changer in this field. By harnessing the power of AI solutions, companies are revolutionizing their supply chain processes and achieving significant improvements in efficiency, accuracy, and cost-effectiveness.
How AI Transforms Supply Chain Management
- Predictive Analytics: AI leverages predictive analytics to foresee potential issues, enabling proactive measures.
- Inventory Management: AI optimizes inventory levels, ensuring that there is neither a surplus nor a shortage of products.
- Demand Forecasting: AI enhances demand forecasting by analyzing historical data, identifying patterns, and accurately predicting demand fluctuations.
- Logistics Optimization: AI streamlines logistics operations, making them more efficient and cost-effective.
Example: Walmart
Walmart, the globally renowned retail giant, utilizes artificial intelligence in its supply chain management to improve productivity and customer satisfaction:
- AI-Driven Demand Forecasting: Machine learning algorithms forecast customer demand, evaluate previous sales data, and manage inventory levels. This ensures product availability, minimizes stockouts, and reduces excess inventory costs.
- Logistics Optimization: AI-powered routing algorithms optimize logistics, enabling faster and more economical delivery.
Walmart’s integration of AI into its supply chain operations exemplifies how cutting-edge technologies enhance decision-making, responsiveness, and overall supply chain resilience in the competitive retail sector.
AI is indeed transforming supply chain management, bringing a host of benefits that drive efficiency and innovation.
Read: Walmart Commerce Technologies Launches AI-Powered Logistics Product
PREDICTIVE MAINTENANCE
In manufacturing, ongoing maintenance of production line machinery and equipment represents a major expense, crucially impacting the bottom line of any asset-reliant production operation. Therefore, predictive maintenance has become a must-have solution for manufacturers. Predictive maintenance uses advanced AI algorithms, including machine learning and artificial neural networks, to predict asset malfunctions. This approach drastically reduces costly unplanned downtime and extends the remaining useful life of production machines and equipment. Predictive maintenance saves businesses valuable time and resources, including labor costs, while guaranteeing optimal manufacturing performance. This is possible through digital twins, sensors, and advanced analytics embedded in manufacturing equipment, enabling predictive maintenance by responding to alerts and resolving machine issues.
Key benefits
- Compared to older data analytics technologies, AI delivers faster, more accurate predictive maintenance.
- By using AI to predict machine failure and maintenance needs, companies can reduce downtime while boosting efficiencies.
- Some of the largest global businesses have deployed AI tools in their predictive maintenance programs, with promising results.
- One key concept in predictive maintenance is the digital twin. A digital twin is a virtual replica of a physical asset that captures real-time data and simulates its behavior in a virtual environment. By connecting the digital twin with sensor data from the equipment, AI for the manufacturing industry can analyze patterns, identify anomalies, and predict potential failures.
Example Some of the world’s largest manufacturers use AI to enhance predictive machine maintenance and improve uptime:
- Global Automaker: Uses AI to inspect and maintain welding robots in its factories. Specifically, it employs computer vision and deep learning to analyze images and videos of robots to spot defects. The AI system recommends parameters and settings for each robot and notifies workers when maintenance or replacement is required. This solution can reduce robot inspection time by 70% and improve welding quality by 10%, according to the automaker.
- GE Aviation: Uses AI to predict the need for maintenance for its jet engines used by airlines and other customers. Approximately 44,000 engines have embedded sensors that feed data to GE monitoring centers in Cincinnati and Shanghai. GE combines this data with physical engine models and environmental details to predict maintenance issues before problems occur. Besides boosting engine reliability, AI has reduced airline maintenance costs and enhanced safety.
Predictive maintenance powered by AI is revolutionizing the manufacturing industry by enhancing operational efficiency, reducing costs, and improving overall reliability and safety.
QUALITY CONTROL
Quality control in manufacturing involves using AI algorithms to notify teams of emerging production faults that could cause product quality issues. Machine-vision tools can find microscopic defects in products at resolutions well beyond human vision by using machine-learning algorithms trained on remarkably small volumes of sample images. When integrated with a cloud-based data processing framework, defects are instantly flagged, and a response is automatically coordinated.
Example: Foxconn implementation of AI in QC
Leading electronics manufacturer Foxconn is a prime example of a business using AI in manufacturing for quality control. Foxconn has enhanced its quality control procedures by incorporating AI and computer vision technologies into its production lines. AI systems quickly and effectively detect flaws in electronic components by examining pictures and videos, ensuring that the goods fulfill strict quality standards. AI in quality control enhances production efficiency and accuracy, allowing firms like Foxconn to produce high-quality goods on a large scale within the rapidly changing electronics sector.
Moreover, AI trends in the manufacturing sector are enhancing predictive quality assurance. By analyzing historical data and real-time sensor data, machine learning (ML) algorithms detect patterns and trends that may indicate potential quality issues. This enables manufacturers to proactively address potential defects and take corrective actions before they impact the final product quality.
Key Benefits of AI in Quality Control
- Increased Accuracy: AI systems can detect even the smallest defects, ensuring higher product quality.
- Speed and Efficiency: Automated inspections are faster than manual checks, increasing overall productivity.
- Cost Reduction: Early detection of defects reduces waste and rework costs.
- Scalability: AI systems can handle large-scale production lines, maintaining consistent quality standards.
Real-World Applications
- Foxconn: Utilizes AI and computer vision to inspect electronic components, ensuring they meet quality standards. AI systems analyze images and videos of products, spotting defects quickly and effectively.
- Automotive Industry: AI-powered systems inspect parts such as engines and transmissions, ensuring they meet stringent quality requirements. Predictive analytics help in identifying potential issues before they become major problems.
- Pharmaceuticals: AI ensures that medicines are produced with the highest quality, analyzing data from production lines to detect any anomalies.
Predictive Quality Assurance with AI
- Pattern Recognition: AI analyzes historical and real-time data to identify patterns that may lead to quality issues.
- Anomaly Detection: AI algorithms detect anomalies in production processes, flagging potential problems early.
- Proactive Measures: By predicting quality issues, manufacturers can take proactive measures to address defects before they affect the final product.
AI-driven quality control is transforming the manufacturing industry, ensuring higher standards, reducing costs, and improving overall efficiency. The integration of AI into quality control processes is a crucial step towards achieving excellence in manufacturing.
Read: Intelligent Manufacturing | Foxconn | Huawei Enterprise
PROCESS OPTIMIZATION
Process optimization is a critical aspect of manufacturing, and artificial intelligence (AI) is a game changer in this domain. AI algorithms can identify patterns, detect anomalies, and make data-driven predictions by analyzing historical data, real-time sensor data, and other relevant variables. This enables manufacturers to optimize operations, minimize downtime, and maximize overall equipment effectiveness (OEE).
How AI Transforms Process Optimization
- Pattern Recognition: AI identifies patterns in production data that humans might miss, allowing for more accurate predictions and better decision-making.
- Anomaly Detection: AI detects anomalies in real-time, flagging potential issues before they escalate into significant problems.
- Predictive Analytics: By analyzing historical and real-time data, AI predicts maintenance needs, equipment failures, and production bottlenecks.
- Operational Efficiency: AI optimizes production schedules, supply chain logistics, and resource allocation, ensuring that manufacturing processes run smoothly.
General Electric (GE) is a prime example of how AI transforms factory performance optimization. GE has integrated AI algorithms into its manufacturing processes to analyze massive volumes of data from sensors and historical records. By using AI, GE can:
- Spot Trends: Identify trends in production data that indicate potential issues or opportunities for improvement.
- Predict Equipment Issues: Forecast probable equipment failures and maintenance needs before they occur.
- Streamline Processes: Optimize manufacturing processes to enhance efficiency and reduce downtime.
Key Achievements of GE’s AI Integration:
- Reduced Downtime: By predicting maintenance needs and potential equipment failures, GE minimizes unplanned downtime, keeping production lines running smoothly.
- Increased OEE: AI helps GE maximize overall equipment effectiveness by ensuring that machinery operates at peak performance.
- Improved Efficiency: Streamlined manufacturing processes result in higher efficiency and productivity, leading to cost savings and better output quality.
Broader Applications and Benefits of AI in Process Optimization
- Supply Chain Management: AI optimizes supply chain logistics by predicting demand, managing inventory levels, and improving delivery schedules.
- Energy Management: AI reduces energy consumption by optimizing power usage and identifying energy-saving opportunities.
- Quality Control: AI enhances quality control by detecting defects in real-time and ensuring that products meet stringent quality standards.
- Customization: AI enables mass customization by adjusting production processes to create personalized products without sacrificing efficiency.
Example of Success:
- Ford Motor Company: Uses AI to optimize its assembly lines, reducing production time and improving product quality.
- Siemens: Employs AI in its factories to enhance production efficiency, leading to significant cost savings and improved output.
- Rockwool: Utilizes Proficy CSense to automate control of complex production processes, including environmental facilities.
- Total EP: Achieved zero unanticipated failures and no downtime by using SmartSignal’s predictive analytics
Read: https://www.gevernova.com/software/products/predictive-analytics
COBOTS: Enhancing Manufacturing with Collaborative Robotics
Cobots, or collaborative robots, are revolutionizing AI-driven manufacturing by working alongside human operators to boost productivity and efficiency. These robots are designed to collaborate with human workers in various tasks, from picking and packing in fulfillment centers to complex assembly processes and quality control inspections.
How Cobots Work
Cobots are equipped with advanced AI systems that enable them to navigate intricate areas, identify objects, and perform tasks with precision and adaptability. They are designed to be safe to work alongside humans, featuring sensors and algorithms that allow them to detect and avoid obstacles, including human workers.
Key Benefits of Cobots in Manufacturing:
- Increased Productivity: By collaborating with human operators, cobots can handle repetitive and physically demanding tasks, allowing human workers to focus on more complex and creative activities.
- Enhanced Efficiency: Cobots can work continuously without fatigue, leading to faster and more efficient production processes.
- Improved Safety: Cobots reduce the risk of workplace injuries by taking on hazardous tasks and working in environments that may be dangerous for humans.
- Cost Reduction: By automating routine tasks, cobots help reduce labor costs and minimize operational expenses.
Example: Amazon
Amazon is a prime example of a company using cobots to enhance its supply chain processes. Here’s how Amazon leverages cobots:
- Order Fulfillment: Amazon’s cobots work in fulfillment centers to pick up and pack items, navigating through the warehouse and collaborating with human workers to ensure efficient order processing.
- Supply Chain Optimization: AI-powered cobots use machine learning to expedite order fulfillment, streamline logistics, and improve overall operations. They handle complex tasks precisely and adaptably, ensuring optimal equipment performance and reducing downtime.
This collaborative strategy exemplifies how cobots and AI work together to create a more productive and agile production environment, where human-machine coordination is key to operational excellence.
Key Benefits:
- Human-Machine Collaboration: Cobots enhance productivity by working alongside human operators, handling tasks that are repetitive, physically demanding, or hazardous.
- Efficiency and Precision: AI-powered cobots perform tasks with high precision, improving the overall efficiency of manufacturing processes.
- Cost and Safety Benefits: Cobots help reduce labor costs and minimize the risk of workplace injuries, creating a safer and more cost-effective production environment.
Read: How Amazon deploys Cobots
WAREHOUSE MANAGEMENT
AI for manufacturing is revolutionizing warehouse management, enhancing efficiency with advanced automation and intelligent data insights. The advent of AI-powered manufacturing solutions and machine learning (ML) in manufacturing has transformed how warehouses operate, improving efficiency, accuracy, and cost savings.
Key AI Applications in Warehouse Management
- Inventory Management: AI algorithms analyze historical sales data, current stock levels, and market trends to accurately predict demand patterns. This enables warehouses to optimize inventory levels, reducing carrying costs and ensuring product availability.
- Automated Guided Vehicles (AGVs): AI-driven AGVs automate the transportation of supplies and finished products within warehouses, streamlining intralogistics operations and enhancing inventory management.
- Order Fulfillment: AI optimizes order processing by managing stock levels, predicting inventory needs, and coordinating the picking and packing process efficiently.
- Warehouse Layout Optimization: AI helps in designing optimal warehouse layouts, improving space utilization, and reducing travel time for picking items.
- Predictive Maintenance: AI systems monitor equipment health and predict maintenance needs, minimizing downtime and ensuring smooth warehouse operations.
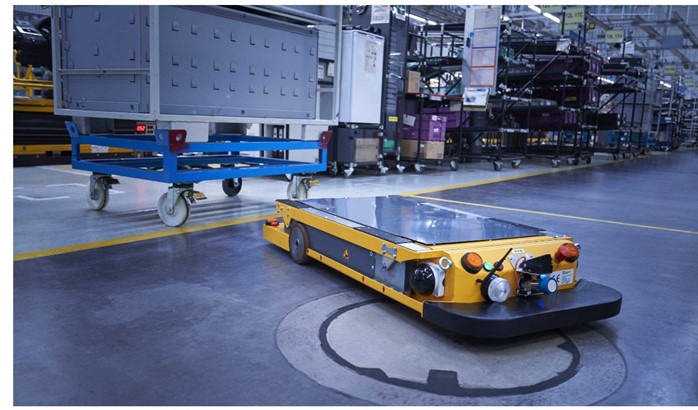
Example: BMW
BMW is a prime example of a company employing AI-driven automated guided vehicles (AGVs) in their manufacturing warehouses to streamline intralogistics operations. Here’s how BMW leverages AI:
- Automated Transportation: AI-powered AGVs follow predetermined paths to transport supplies and finished products, automating the transportation process and enhancing inventory management.
- Improved Visibility: AI systems provide real-time visibility into inventory levels, enabling better decision-making and efficient resource allocation.
BMW’s integration of AI into warehouse management demonstrates how cutting-edge technologies can enhance operational efficiency, reduce costs, and improve overall productivity.
Benefits of AI-Powered Warehouse Management
- Increased Efficiency: AI automates routine tasks, reducing manual intervention and speeding up warehouse operations.
- Cost Savings: Optimized inventory levels and automated processes lead to significant cost savings.
- Enhanced Accuracy: AI ensures precise inventory management, minimizing errors and stock discrepancies.
- Scalability: AI solutions can easily scale to accommodate increasing demand and expanding operations.
- Improved Customer Satisfaction: Efficient order fulfillment and accurate inventory management result in timely deliveries and satisfied customers.
The integration of AI in the manufacturing market has brought significant advancements to warehouse management. From inventory optimization to streamlined order fulfillment, AI-powered manufacturing and ML solutions are transforming warehouses, making them more efficient and responsive to market demands.
Read: BMW Group is making logistics robots faster and smarter
ASSEMBLY LINE OPTIMIZATION
Assembly line optimization using Artificial Intelligence (AI) is revolutionizing production processes by enhancing accuracy, efficiency, and flexibility. By analyzing past performance metrics and real-time sensor data, machine learning algorithms can improve workflow, reduce downtime, and enable predictive maintenance. Here’s a deeper look at how AI is transforming assembly lines:
Key Components of AI-Driven Assembly Line Optimization
- Machine Learning Algorithms: These algorithms analyze historical data and real-time sensor inputs to identify patterns, predict equipment failures, and optimize production schedules.
- Predictive Maintenance: AI predicts when equipment is likely to fail, allowing for timely maintenance that prevents unplanned downtime and extends the lifespan of machinery.
- Computer Vision Systems: AI-driven computer vision can detect flaws or anomalies in products with greater precision than human inspectors, ensuring higher product quality.
- Intelligent Automation: By adjusting production parameters in response to demand variations, intelligent automation reduces waste and improves resource utilization.
- Data-Driven Decision Making: AI turns assembly lines into data-driven, flexible environments through continuous learning and adaptation, ultimately boosting output, lowering costs, and maintaining high manufacturing standards.
Example: Volkswagen
Volkswagen is a prominent example of a business using AI to optimize assembly lines in the Industry 5.0 era. Here’s how Volkswagen leverages AI:
- Sensor Data Analysis: Volkswagen uses machine learning algorithms to analyze sensor data from the assembly line. This analysis helps forecast maintenance requirements and streamline operations.
- Enhanced Efficiency and Quality: By integrating AI-driven solutions, Volkswagen improves the efficiency and quality of its production processes. This proactive approach minimizes downtime, reduces maintenance costs, and ensures optimal equipment performance.
Key Benefits of AI in Assembly Line Optimization:
- Improved Accuracy: AI enhances the precision of production processes, reducing the likelihood of errors and defects.
- Increased Efficiency: Automated systems can work continuously without fatigue, speeding up production and improving overall efficiency.
- Enhanced Flexibility: AI allows for quick adjustments to production parameters in response to changes in demand or other variables.
- Cost Reduction: By optimizing resource utilization and reducing waste, AI helps lower operational costs.
- Sustainability: AI-driven processes often result in more efficient use of materials and energy, contributing to environmental sustainability.
Broader Implications and Future Directions
AI-driven assembly line optimization is a cornerstone of Industry 5.0, where human-centric, sustainable, and resilient production systems are the focus. As AI technologies continue to evolve, we can expect further advancements in:
- Real-Time Analytics: Enhanced real-time data analysis will provide even more precise insights and allow for instant decision-making.
- Robotic Integration: Increased collaboration between human workers and AI-powered robots (cobots) will further streamline assembly line operations.
- Adaptive Manufacturing: AI systems will become more adaptive, learning from every process and continuously improving production efficiency.
In summary, AI-driven assembly line optimization is transforming manufacturing by improving accuracy, efficiency, flexibility, and sustainability. Companies like Volkswagen exemplify how AI can enhance production operations, reduce costs, and ensure high-quality output.
Read: 5 ways Volkswagen is using AI [Case Study] [2024] – DigitalDefynd
ORDER MANAGEMENT
Using artificial intelligence (AI) in order management involves optimizing and streamlining the entire order fulfillment process. AI analyzes past data, consumer preferences, and market trends using machine learning algorithms to estimate demand accurately. This capability allows for automatic order processing, inventory optimization, and dynamic pricing adjustments. Additionally, AI enhances fraud detection, reducing the risks associated with fraudulent orders.
AI solutions in manufacturing increase the overall effectiveness of order management systems, speed up decision-making, and ensure a more responsive and customer-centric approach to order fulfillment. By automating repetitive operations and providing data-driven insights, AI helps companies across various industries achieve significant improvements in their order management processes.
Example: IBM Watson
One practical example of AI in manufacturing is the IBM Watson Order Optimizer. This AI/ML-powered solution analyzes past order data, customer behavior, and other external factors. By leveraging these insights, the system optimizes order fulfillment processes, dynamically adjusts inventory levels, and recommends efficient order routing strategies. This helps companies reduce expenses, increase customer satisfaction, and improve order management efficiency.
Key Features and Benefits of IBM Watson Order Optimizer:
- Demand Forecasting: AI algorithms predict demand accurately, ensuring optimal inventory levels and reducing carrying costs.
- Dynamic Inventory Management: AI adjusts inventory levels based on real-time data, preventing overstocking or stockouts.
- Efficient Order Routing: AI recommends the best routing strategies for orders, optimizing delivery times and reducing logistics costs.
- Fraud Detection: AI systems detect and mitigate fraudulent orders, enhancing security and reducing financial losses.
- Improved Customer Satisfaction: Streamlined order fulfillment processes lead to faster delivery times and higher customer satisfaction rates.
Example of Success:
- IBM Watson: The IBM Watson Order Optimizer has been successfully implemented by various companies, showcasing the transformative potential of integrating AI into manufacturing. This solution helps optimize intricate order fulfillment processes, enhancing supply chain responsiveness and overall efficiency.
AI-powered order management systems are transforming how companies handle their supply chains, ensuring better efficiency, accuracy, and customer satisfaction. By leveraging advanced AI solutions like IBM Watson, businesses can stay competitive in the fast-paced manufacturing industry.
Read: IBM watsonx Orders
FORECASTING WITH AI
In today’s rapidly evolving technological landscape, managing technical risks has become a critical concern for organizations across various industries. AI has the capability to process vast amounts of data at remarkable speeds, uncovering patterns and insights that would be difficult for humans to detect. This technology is being applied to transform business forecasting by integrating advanced AI techniques with traditional financial practices, resulting in higher levels of accuracy and efficiency.
Evolution of Forecasting
Historically, forecasting, budgeting, and variance analysis have relied on manual processes and historical data. However, with the increasing complexity and volatility of markets, there is a growing demand for more agile, data-driven approaches. AI leverages sophisticated algorithms and machine learning to process information from diverse sources, identifying hidden patterns and providing predictions that surpass human abilities.
Applications of AI in Forecasting
- Supply Chain: Retail Inventory Management
- Retail chains leverage AI to optimize inventory levels across their stores. AI analyzes sales data, foot traffic, and external factors like weather. AI systems can also provide recommendations on replenishment quantities and timing. This minimizes overstocking and understocking issues, leading to improved profitability and customer satisfaction.
- Operations: Energy Consumption Prediction
- Utility companies employ AI to forecast energy consumption patterns. By considering historical usage, weather forecasts, and economic indicators, AI models can predict peak demand periods and engage users to optimize their energy consumption. This helps prevent power shortages during high-demand periods and enhances overall grid stability.
- HR: Predictive Performance Modeling
- Several companies are successfully leveraging predictive performance modeling to enhance their HR practices:
- Trakstar: This platform provides tools for performance management that include predictive analytics to help organizations identify top talent.
- Visier: Known for its workforce analytics solutions, Visier enables organizations to visualize performance trends and make strategic decisions based on predictive insights.
- Akkio: This AI-driven platform offers predictive analytics capabilities that help HR teams forecast employee performance and optimize talent management strategies.
- Several companies are successfully leveraging predictive performance modeling to enhance their HR practices:
Benefits of AI in Forecasting
- Increased Accuracy: AI’s ability to analyze vast datasets leads to more accurate predictions and better decision-making.
- Efficiency: Automation of forecasting processes saves time and reduces the potential for human error.
- Agility: AI allows businesses to quickly adapt to changing market conditions and make data-driven decisions.
- Cost Savings: Optimized inventory management and efficient resource utilization result in significant cost savings.
AI is transforming the way businesses approach forecasting, providing them with the tools to navigate an increasingly complex and volatile market landscape. By integrating AI into forecasting processes, companies can achieve higher levels of accuracy, efficiency, and agility, ensuring they stay competitive in today’s fast-paced environment.
Read: AI Forecasting Techniques: How They Work, Models & Challenges
CONNECTED FACTORIES
Connected factories are prime examples of how artificial intelligence (AI) can be incorporated into production processes to build intelligent, networked ecosystems. Leveraging AI in manufacturing helps evaluate real-time data from machinery, anticipate maintenance requirements, streamline operations, and reduce downtime using IoT sensors.
Key Features of Connected Factories
- Real-Time Data Evaluation: AI analyzes data from machinery in real-time, allowing for immediate identification of issues and optimization of operations.
- Predictive Maintenance: AI algorithms predict when maintenance is required, reducing unplanned downtime and extending equipment lifespan.
- Streamlined Operations: Automated systems optimize production schedules, manage inventory, and coordinate supply chain activities.
- Machine-to-Machine Communication: IoT sensors enable effective communication between machines, allowing for quick adjustments to production processes in response to demand changes.
- Predictive Analytics: AI enhances decision-making by using predictive analytics to forecast trends and potential disruptions, ensuring seamless operations.
Benefits of AI-Driven Connected Factories
- Cost Reduction: By minimizing downtime and optimizing resource utilization, AI-driven connected factories significantly reduce operational costs.
- Increased Efficiency: Automation and real-time data analysis improve overall operational efficiency and productivity.
- Enhanced Flexibility: Connected factories can quickly adapt to changing circumstances, ensuring consistent production quality and meeting market demands.
- Improved Decision-Making: Predictive analytics provide valuable insights that enable proactive decision-making and risk management.
Example: General Electric (GE)
General Electric (GE) is a notable example of a company leveraging AI-based connected factories. GE uses its Predix platform to integrate AI with the Internet of Things (IoT) in manufacturing. This system allows GE to monitor equipment health, predict maintenance needs, and optimize production lines. Key features of GE’s Predix platform include:
- Equipment Health Monitoring: Continuous monitoring of machinery health to detect issues early.
- Predictive Maintenance: Forecasting maintenance needs to prevent unexpected failures.
- Data Analysis: Utilizing data from IoT sensors to optimize production processes and reduce downtime.
Key Achievements:
- Reduced Downtime: GE’s Predix platform has significantly reduced unplanned downtime, ensuring smoother production operations.
- Enhanced Efficiency: The integration of AI and IoT has boosted factory efficiency through data-driven insights and automated processes.
Connected factories exemplify how AI can create data-driven, adaptive manufacturing ecosystems that respond quickly to changing circumstances. By leveraging AI and IoT technologies, companies like GE are transforming their production processes, improving efficiency, and maintaining high standards in manufacturing.
STREAMLINED DAILY TASKS AND DOCUMENTATION WITH AI
One impactful application of AI and Machine Learning (ML) in manufacturing is Robotic Process Automation (RPA) for paperwork automation. Traditionally, manufacturing operations involve a lot of paperwork, such as purchase orders, invoices, and quality control reports. These manual processes are time-consuming, error-prone, and can result in delays and inefficiencies.
How AI and RPA Streamline Paperwork
- Automated Data Extraction: AI-driven bots can automatically extract data from documents, classify and categorize information, and integrate it into appropriate systems. This helps in streamlining processes and enhancing accuracy.
- Conversational AI: Implementing conversational AI in manufacturing allows companies to automate communication-related tasks, reducing the burden on human workers and speeding up response times.
- Process Automation: RPA bots handle repetitive and rule-based tasks, ensuring accuracy and productivity in the manufacturing process.
Example: Whirlpool
Whirlpool utilizes RPA to automate its manufacturing processes, particularly on the assembly line and material handling tasks. Here’s how Whirlpool leverages RPA:
- Assembly Line and Material Handling: RPA bots carry out repetitive and rule-based tasks, guaranteeing accuracy and productivity in the manufacturing process.
- Quality Control Inspections: Whirlpool employs RPA bots for quality control inspections, utilizing automation to improve uniformity and accuracy in evaluating the finished product.
By automating these processes, Whirlpool streamlines its operations and maintains a high standard of product quality.
Key Benefits of Streamlined Paperwork with AI and RPA
- Increased Accuracy: Automation reduces the likelihood of errors that are common in manual processes.
- Time Savings: Automated processes are faster than manual ones, leading to quicker order processing and invoicing.
- Cost Reduction: Reducing manual labor for paperwork tasks leads to significant cost savings.
- Enhanced Efficiency: Streamlined processes improve overall operational efficiency and productivity.
Implementing AI and RPA for paperwork automation in manufacturing not only reduces manual errors and saves time but also ensures that processes are more efficient and cost-effective. Companies like Whirlpool are leading the way in showcasing the benefits of these technologies in maintaining high standards of product quality and operational excellence.
Read: Whirlpool’s 10 Steps To Digital Transformation In A/R SSCs
Conclusion
As we navigate the dynamic landscape of modern manufacturing, the integration of AI and advanced technologies is proving to be a game-changer. From connected factories and predictive maintenance to robotics, process optimization, order management, and streamlined paperwork, the transformative power of AI is evident across every facet of the industry. Companies like General Electric (GE) and Whirlpool are leading the charge, showcasing how AI can enhance efficiency, accuracy, and overall productivity.
AI-driven solutions are not only optimizing traditional processes but also paving the way for the future of manufacturing with Industry 5.0. This new era emphasizes human-centric, sustainable, and resilient production systems. By leveraging AI technologies, manufacturers can achieve higher levels of performance, reduce costs, and maintain high standards of quality.
The seamless integration of AI with other advanced technologies like IoT, machine learning, and robotic process automation is creating intelligent, adaptive ecosystems that respond swiftly to changing demands and market conditions. The examples of Amazon, IBM Watson, and Volkswagen demonstrate the broad applicability and significant benefits of AI across various industries.
As we move forward, the continued advancement and adoption of AI technologies will undoubtedly lead to further innovations and improvements in manufacturing processes, ensuring that companies remain competitive and capable of meeting the ever-evolving needs of their customers.
References
1.AI in Manufacturing: Use Cases and Examples
2.Industry 5.0: Prospect and retrospect – ScienceDirect
6. Understanding Robotic Process Automation (RPA) | Pega
9. Integrated SCARA Robots Redefine Precision | Schneider Electric
10. Using AI in Predictive Maintenance: What You Need to Know
11. THE ROLE OF ARTIFICIAL INTELLIGENCE IN FORECASTING AND MANAGING TECHNICAL RISKS
12. Companies Using Ai For Forecasting | Restackio
13. Robots and its types for industrial applications – ScienceDirect
14. Artificial Intelligence in Material Science: Advances – Google Books
I hope this blog has provided you with valuable insights into the transformative power of AI in manufacturing and how it is driving the next industrial revolution. If you have any further questions or need more information, feel free to reach out us. Contact: info@mykanaq.com
Author: Haseena Begum, Supply chain team, KanaQ Innovations, India
All inputs of this blog are from open source research and added all references